How Artificial Intelligence is powering Airly’s forecasting capabilities
Recent Posts
Contact us
Airly provides the comprehensive air quality monitoring solution for local governments and businesses. Contact our Team and get access to advanced analytics and insights about air quality in your area.
Monika
Jankowska
Dimal
Luta
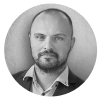
Mateusz
Koczwara
Aleksandra
Fraś-Zdeb